Hierarchically
organized serial patterns. One prediction from the rule-learning
view is that a highly organized, hierarchically structured sequence should
be easier to learn than a sequence having little or no higher-order
structure. We designed several studies to explore whether pattern
structure would determine the ease or difficulty of learning long and
elaborate patterns.
In one
experiment (Fountain et al., 1995b),
we tested whether pattern structure described as “runs” (e.g.,
1-2-3-4-5-…) or “trills” (e.g., 1-2-1-2-1-…) would determine the ease or
difficulty of anticipating a final sequence item that either conformed to
the implied structure of the sequence or violated pattern structure. Rats
received patterns having either perfect structure or one sequence element
(the last in the series) that violated an otherwise perfect structure:
"Perfect Runs" *************** 567
678 781 812 ...
"Violation Runs" *************** 567
678 781 818 ... (violation element indicated)
"Perfect Trills" 121 232 343 454
565 676 787 818 ...
"Violation Trills" 121 232 343 454
565 676 787 812 ... (violation element indicated)
A 1-s ITI was used
except where spaces indicate 3-s phrasing cues. [Note once again that
Lever 1 is immediately to the right of Lever 8, so that, for example, a
6-7-8-1-2 sequence would be a quite natural "run" series.] As shown in
Figure 3, high error rates were observed in acquisition on the
violation trial (the last trial of the pattern) for both Violation Runs
and Violation Trills patterns, despite the fact that one view might
predict generalization of associations from other parts of the pattern
should have predisposed animals to learn the violation patterns easily.
For example, in the Violation Trills pattern a correct response on lever 1
should always predict that the next response should be to lever 2, yet
rats had great difficulty learning to respond on Lever 2 on the last trial
of the pattern but not the second trial of the pattern. No comparable
errors were observed for the Perfect Runs or Perfect Trills patterns. An
alternative view is that rats learned about the highly repetitive
structure of the sequence that resulted in highly repetitive patterns of
response to learn the sequence even when doing so produced errors at the
violation element, even though these errors might have been avoided by
adopting another strategy. CF1 mice show the same pattern of results as
rats when learning the perfect and violation runs patterns described here(Fountain et al., 1999).
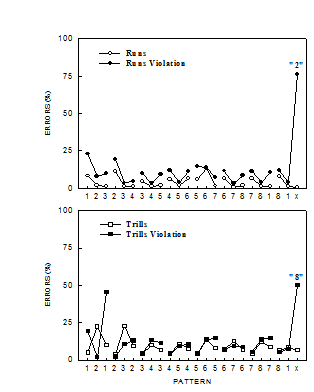
|
Figure 3.
Rats' mean
percentage of pattern tracking errors for hierarchical (top panel) and
linear (bottom panel) patterns as a function of the 24 items of the
patterns collapsed across 7 days of training. “Runs” versus “trills”
structure predicted difficulty and types of errors observed for the
final violation element of the violation patterns (Fountain & Rowan,
1995b). |
In another
set of studies, we tested whether pattern structure would determine the
ease or difficulty of pattern learning by developing patterns with
hierarchical structure, then reordering chunks of the pattern to produce
“linear” structure, that is, a sequence of unrelated chunks. The
Hierarchical (H) and Linear (L) patterns were:
H Pattern: *************** 567 876 765 654
543 432...
L Pattern: 123 234 543 456 567 876 765
654 345 432...
For both groups, the digits
indicate the clockwise position of the correct response on successive
trials and spaces indicate brief pauses.
The
completely nested H pattern is described by a simple hierarchical rule
structure: elements within 3-element chunks are related by first-order
rules, chunks within the first and second halves of the pattern,
respectively, are related to each other by second-order rules, and the
first half of the pattern is related to the second half of the pattern by
a third-order rule. A formal description of this pattern is (M(T+14(T+12(1)))),
where “1” refers to the
starting lever, T+n represents a “transpose” rule (i.e.,
to move n units in the indicated direction, where + indicates
clockwise), M represents a “mirror image” rule, and superscripts reflect
the number of repeated applications of the rule that are required.
Because of the nested structural organization, the second-order T+1
rule applies a “+1” rule to each item in the first chunk to generate the
second chunk, and so on. The third-order M rule produces a “mirror image”
(a more complex form of a “reverse” rule) of the first half of the pattern
to generate the entire second half of the pattern.
The
incompletely nested L pattern was generated by exchanging the two
underlined 3-element chunks in the H pattern. In so doing, however, it
should be noted that all pairwise associations were maintained; rats were
always required to press a lever immediately to the left or right of the
last correct response in both patterns, and the number of transitions from
a given lever to any other was conserved across patterns. In this
structure, elements within any chunk are related by a rule, but chunks are
not related to each other in any systematic way. A formal description of
this pattern is (T+1(T+12(1))) - (T-12(5))
- (T+1(T+12(4))) - (T-12(T-12(8)))
- (T+12(3)) - (T-12(4)), where
T+n and T-n represent rules indicating
to “transpose” clockwise and counter-clockwise, respectively. Dashed
lines indicate connections that must be learned by non-hierarchical rules
or non-rule-based associations between rule-governed chunks or chunk
subsets. Note that this complicated structure resulted from changing the
serial positions of only 4 of 30 pattern elements compared to the H
pattern.
The results
showed that, for rats, pattern complexity predicted pattern learning
difficulty (Fountain & Rowan, 1995a). The formally simpler H pattern was
easier to learn than the formally more complex L pattern. In addition,
rats in H were sensitive to the hierarchical structure of their pattern.
For rats, as in humans, in the H pattern groups, the difficulty of
learning to respond appropriately on any trial was a function of the
hierarchical level of the rule required to predict the item. Figure
4
shows rats' group mean element-by-element error rates for Week 1 of the
experiment for the H group (top panel) and the L group (bottom panel). As
shown in Figure 4, during Week 1, rats produced significantly more
errors on the first trial of Chunks 1 and 6 than on all other trials.
These trials corresponded to the highest-order rule transitions in the
pattern structure (i.e., third-order rule transitions). Fewer errors were
observed on the first trial of other chunks; these trials corresponded to
second-order rule transitions. The fewest errors occurred within chunks,
where trials corresponded to first-order rule transitions. Thus, in the
completely hierarchical pattern, the difficulty of learning to respond
appropriately on any trial was a function of the hierarchical level of the
rule required to predict the item.
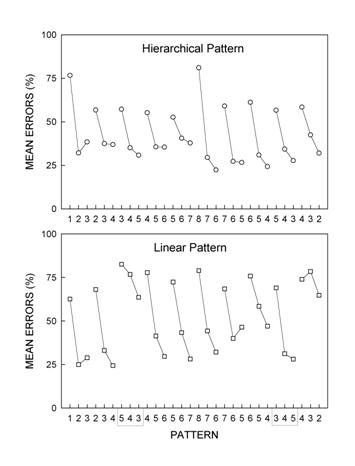 |
Figure 4.
Rats' mean percentage of pattern tracking errors for hierarchical
(top panel) and linear (bottom panel) patterns as a function of the
30 items of the patterns. Mean percentage of errors are shown for
the first week of training. Pattern complexity predicted pattern
learning difficulty and features of pattern structure predicted the
kinds of “intrusion” errors observed (Fountain & Rowan, 1995a).
|
Rats in L
did not show the 3-level hierarchical pattern of errors observed for H
rats. L rats found trials within chunks easier than the first trial of
each chunk, but their response to the first trial of chunks was
disorganized. That is, L rats, unlike H rats, showed no differential
response for the first trial of Chunks 1 and 6 (corresponding to
third-order rule transitions in the completely nested pattern) versus the
first trials of other chunks (corresponding to second-order rule
transitions in the completely nested pattern). However, error rates
for the second and third elements of each chunk (with the exception of the
second element of Chunks 3 and 10) were significantly lower than for the
first element of each chunk. These results support the view that L rats
were sensitive to the actual pattern structure; they recognized that
elements within a given chunk were related by a single rule, but that
chunks were somewhat haphazardly arranged.
In the
hierarchical versus linear structure experiment just described, rats
demonstrated sensitivity to multi-level hierarchically-organized pattern
structure. Rats found learning completely nested hierarchical patterns
easier than learning less organized patterns even when pairwise
associations and pattern length were conserved across patterns. In
another study from the same series (Fountain et al., 1995a),
a 3-level hierarchy was easier to learn than a 4-level hierarchy when
pattern length was conserved across patterns. As a rule, then, pattern
complexity was a better predictor of acquisition difficulty in these
studies than was pattern length. These acquisition results alone are
strong evidence that pattern organization, that is, pattern complexity,
was the primary determinant of pattern difficulty, as argued by a
rule-learning view of sequential learning.
Interleaved serial patterns. One question of significance for animal sequential learning research is
whether animals are constrained to learn sequences on the basis of
pair-wise associations between successive elements, for example, as in
chaining (Skinner, 1934).
A significant body of evidence suggests that animals are able to be more
flexible in representing sequential events, conceivably by coding
hierarchical representations characterized by relations for nonadjacent
events.
The mechanisms of learning involving nonadjacent events are not
well-understood. Terrace(1987),
for example, indicated that little evidence existed that animals are able
to spontaneously reorganize sequentially-presented items into chunks not
presented by the experimenter. As Terrace noted, such processes are
readily observed in human free-recall (Tulving, 1962).
Additionally, it should be noted that chunking of nonadjacent items in
human serial-pattern learning has been studied extensively using patterns
of letters and digits (e.g., Hersh, 1974).
We have previously shown that rats, when presented a sequence of reward
quantities, can spontaneously sort quantities from nonadjacent serial
positions into chunks to facilitate learning (Fountain & Annau, 1984).
A comparable strategy in humans would be to learn the pattern
2555455565558 by sorting pattern elements into 555 chunks and a 2468
chunk. Other work also supports the view that rats have this capacity.
For example, Capaldi and Miller (1988) have shown that rats can keep count
of different kinds of rewards by chunking nonadjacent items in series into
different food categories. In two recent studies in our laboratory
(Fountain, Rowan, & Benson, Jr., 1999),
rats learned either a structured (ST) or unstructured (UNST) sequence
interleaved with elements of a repeating (R) sequence in one experiment or
an alternation (A) sequence in another experiment. The question was
whether rats would learn the interleaved subpatterns at different rates as
a function of subpattern complexity.
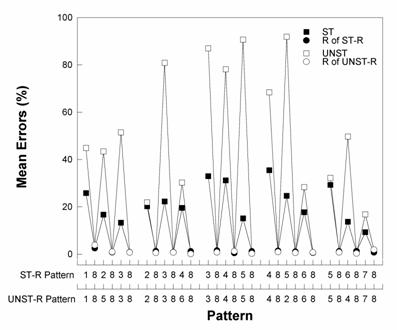 |
Figure 5. Group mean element-by-element
errors for the ST-R and UNST-R interleaved patterns averaged across
Week 3 of training (Fountain, Rowan, & Benson, 1999). |
The first experiment sought to determine
whether rats would show signs of being sensitive to the organization of
nonadjacent items from interleaved subpatterns when one subpattern was a
composed of simple, repeating element and the second subpattern was either
highly structured or not. For rats in the Structured (ST) subpattern
condition, a *************** 567 subpattern was interleaved with a
Repeating (R) subpattern, 888 888 888 888 888, resulting in the ST-R
pattern that rats were required to learn:
182838 283848 384858 485868 586878.
For rats in the
Unstructured (UNST) subpattern condition, a 153 236 345 426 547 subpattern
was interleaved with the same R subpattern to create the UNST-R pattern in
the same manner. For both patterns, integers represent the clockwise
position of correct levers in the octagonal chamber on successive trials
and spaces represent pauses that served as phrasing cues.
Acquisition of the interleaved structured
pattern (i.e., ST-R) was significantly faster than for the interleaved
unstructured pattern (i.e., UNST-R). The unstructured pattern was
generated by exchanging only two pairs of elements in the structured
pattern, as described above. In so doing, however, all pair‑wise
associations in the interleaved patterns were maintained because all of
the relocated items were preceded by “8” trials. Nevertheless, Figure
5 shows that the effects of disrupting pattern structure were apparent
throughout the pattern. This was so even in the third (middle) chunk that
was not altered in producing the unstructured pattern; rats found this
chunk, 384858, harder to learn in the context of the UNST-R pattern than
in the ST-R pattern.
In the second experiment, rats learned two
interleaved sequences where both were created from sets composed of more
than one element. As before, longer patterns were composed of two
interleaved subpatterns; either a structured or unstructured subpattern
was interleaved with a subpattern of two alternating elements. For one
group of rats, the structured (ST) subpattern, 1 2 3 4 5 6, was
interleaved with the alternating (A) subpattern, 7 8 7 8 7 8 to create the
ST-A pattern. For another group of rats, the unstructured (UNST)
subpattern, 1 5 3 4 2 6, was likewise interleaved with the same
alternating subpattern to produce the UNST-A pattern. Note that the
unstructured subpattern was generated by exchanging two items of the
structured subpattern. Rats learned the subpatterns of their interleaved
patterns at different rates both within and between pattern groups. As
predicted based on subpattern structure, in the case of the UNST-A
pattern, the A subpattern was acquired faster than the UNST subpattern.
The A subpattern would be expected to be acquired faster because it is
formally simple whereas the UNST subpattern has little structure.
Based on similar reasoning, it was expected that the ST subpattern should
be easier to learn than the UNST subpattern, and this result was
obtained. In the case of the ST-A pattern, since both subpatterns were
structured, it might be difficult to predict in advance based on
subpattern structure alone whether rats should find either the ST or A
subpattern easier to learn than the other. However, if structural
complexity is equated (i.e., if the same number of rules are needed to
describe subpattern structure), rats might show the same predisposition
that humans do (Kotovsky & Simon, 1973)
to detect repeating items before other structural features of patterns.
In fact, evidence for the latter assertion was obtained in this
experiment. Rats in the ST-A pattern group showed better acquisition for
A with its repeating “7” and “8” elements than ST subpatterns of their
interleaved pattern despite the fact that both ST and A subpatterns have
simple structure that can be described by a single rule (viz., a “+1” rule
for the 123456 ST subpattern versus an “alternate” rule for the 787878 A
subpattern). The results of differential acquisition of ST and UNST
subpatterns support the notion that accurate performance on these
interleaved subpatterns was dependent on a mnemonic representation
characterized by relations for nonadjacent events.
The results indicate that rats are sensitive to the organization of
nonadjacent elements in serial patterns and that they can detect and sort
structural relationships in interleaved patterns. Pattern and subpattern
structure appear to drive how animals sort, chunk together, and represent
nonadjacent pattern elements that are related by common rules or
features.
|